Intel quietly acquires private 5G software provider Ananki
Intel has acquired private 5G network provider Ananki, several months after the startup spun out of the non-profit Open Networking Foundation (ONF) to commercialize open-source network technologies.
The acquisition was confirmed Monday on LinkedIn by Guru Parulkar, PhD, who was co-founder and CEO of Ananki and executive director of the Open Networking Foundation.
–>His ONF successor was not disclosed, despite my LI comment enquiring about it.
Intel declined to comment on the Ananki acquisition and instead only confirmed a development that Parulkar said was related: that the ONF’s development team has joined Intel’s Networking and Edge Group. Intel’s statement echoed a quote provided by top Intel networking executive and former Stanford Professor Nick McKeown, PhD in a press release published by the non-profit. McKeown was previously a part-time Intel Senior Fellow who joined the company after its 2019 acquisition of Barefoot Networks, which he co-founded.
“The addition of these developers will support [Intel’s Network and Edge Group’s] mission to drive the shift toward software-defined and fully programmable infrastructure – from the cloud, through the Internet and 5G networks, all the way out to the Intelligent Edge. Intel intends to continue to support and contribute to ONF’s open-source efforts,” an Intel spokesperson said. No financial terms were disclosed.
Ananki provides an open-source, software-defined service that aims to make private 5G networks “as easy to consume as Wi-Fi” for enterprises working on so-called Industry 4.0 projects. This involves connecting a variety of things, including cameras, sensors, robots, and autonomous vehicles, over high-speed networks in various settings, from factories to retail stores.
Ananki has a diverse range of products, including a SaaS-based 5G software stack, small cell radios, SIM cards, and a dashboard for monitoring and analyzing network activity. These are provided through a subscription-based service that charges organizations based on how much 5G coverage they need.
Source: Ananki
If Intel continues to offer Ananki’s products as a subscription service, it would fall in line with the semiconductor giant’s plan to buoy hardware sales with a significant increase in software revenue, as The Register has previously reported. Less than two weeks ago, The Register reported that Intel plans to offer the cloud optimization software of Granulate, another startup it plans to acquire, in Xeon CPU sales pitches.
The Ananki transaction is part of a broader effort by the Open Networking Foundation to support the increasing commercialization of its open-source, software-defined networking technologies, which it originally developed with the financial support of its more than 100 members. Those include Intel as well as several other prominent tech companies, such as AMD, AT&T, Broadcom, Cisco, Google, Microsoft, Nvidia, and T-Mobile.
The Open Networking Foundation said this new commercialization shift involves open-sourcing the entirety of its production-ready software, which includes private 5G, SD-RAN, SD-Fabric and SD-Core technologies that serve as the basis of Ananki’s products. The nonprofit has also made its software-defined broadband and P4 programmable network technologies available as open source.
“We have built platforms that naysayers said were doomed to fail, we’ve proven what’s possible, and today a number of our platforms have been deployed in production networks and others are now production ready and expected to be broadly adopted,” said Parulkar, who is now vice president of software within Intel’s Network and Edge Group.
The ONF seems to want to move development from internal open source teams to member organizations. As such, the nonprofit is transitioning a majority of its development team to Intel’s Network and Edge Group, which is also the new home of Ananki.
References:
https://www.theregister.com/2022/04/12/intel_ananki_5g/
https://www.intel.com/content/www/us/en/edge-computing/what-is-the-network-edge.html
https://networkbuilders.intel.com/events2022/big-5g-event
ONF Enters a New Era Focused on Growing Adoption and Community for its Leading Open Source Projects
Global Wi-Fi 6 market forecast to grow from $11.5B in 2022 to $26.2B by 2027; CAGR=17.9%
According to a new research report “Wi-Fi 6 Market Global Forecast to 2027,” published by MarketsandMarkets™, the global Wi-Fi 6 market size is expected to grow from $11.5 billion in 2022 to $26.2 billion by 2027, at a Compound Annual Growth Rate (CAGR) of 17.9% during the forecast period.
……………………………………………………………………………………………………………………………………
Editor’s Note:
Wi-Fi 6 is an acronym for the IEEE 802.11ax standard. Prior to the release of Wi-Fi 6, Wi-Fi standards were identified by version numbers ranging from 802.11b to 802.11ac.
…………………………………………………………………………………………………………………….
The managed services Wi-Fi 6 market segment is expected to grow at a higher CAGR than enterprise or consumer Wi-Fi 6 during the forecast period. Managed Service Providers (MSPs) offer are third–party IT service providers that remotely manage the IT infrastructure and systems of clients for backup and recovery of business–critical data. These service providers carry out 24/7 remote monitoring of Wi–Fi 6 networks for their commercial clients. Enterprises opt for managed services to overcome the challenges of budget constraints and technical expertise as managed service providers have skilled human resources, infrastructure, and industry certifications. They offer services to monitor and manage hardware devices and manage the availability and the performance of networks. They also ensure smooth operations and security of networks. The growth of the Wi–Fi 6 market is being driven by the increasing reliance by businesses on the use of IT to improve business productivity, coupled with a continuing rise in demand for specialized MSPs and cloud–based managed Wi–Fi 6 services.
Asia Pacific (APAC) region to record the highest growing region in the Wi-Fi 6 Market. Important countries include Australia, Japan, Singapore, India, China, and New Zealand. The region is expected to witness the fast-paced adoption of Wi-Fi 6 software. The Asia Pacific region is estimated to be the fastest-growing Wi-Fi 6 Market owing to the rise in the adoption of new technologies, high investments for digital transformation, the rapid expansion of domestic enterprises, extensive development of infrastructures, and increasing GDP of various countries. Rapidly growing economies, such as China, Japan, Singapore, and India, are implementing Wi-Fi 6 solutions across multiple business processes to provide effective solutions.
Key and innovative vendors in the Wi-Fi 6 market are:
Cisco Systems (US), Intel Corporation (US), Huawei Technologies (China), NETGEAR (US), Juniper Networks (US), Broadcom (US), Qualcomm Inc. (US), Extreme Networks (US), Ubiquiti Networks (US), Fortinet Inc. (US), Aruba Networks (US), NXP Semiconductors (Netherlands), AT&T (US), Cambium Networks (US), D-Link Corporation (China), Alcatel-Lucent (US), TP-Link (China), MediaTek (China), Telstra (Australia), Murata (Japan), Sterlite Technologies Limited (India), Celeno (Israel), H3C (China), Senscomm Semiconductor (China), XUNISON (Ireland), Redway Networks (UK), VSORA (France), NEWRACOM (US), WILUS Group (South Korea), Federated Wireless (US).
References:
Cignal AI: Datacom optical component revenue +27% to reach $4.7B in 2021
Cignal AI reports that total revenue for optical components, a category that includes optical transceivers, grew 15% in 2021. Components for datacom optical network applications led the way, growing 27% to account for $4.7 billion of the total $14.5 billion component sales registered for the year within the datacom, telecom, industrial, and consumer markets, the market research firm states in its latest Optical Components Report.
A transition by large cloud service providers and some enterprise network operators toward 400-Gbps transmission helped spur this growth. For example, 1.8 million QSFP-DD and OSFP datacom modules shipped during 2021, most of which were DR4 format. Meanwhile, more than 60,000 400G pluggable coherent modules shipped at the same time, with QSFP-DD ZR devices accounting for the majority.
“The transition to 400GbE is well underway, and pluggable coherent 400Gbps technology is revolutionizing the design of the optical networks that connect datacenters,” said Scott Wilkinson, Lead Optical Component Analyst at Cignal AI. “400Gbps speeds will drive spending and bandwidth growth both inside and outside the datacenter in 2022,” Scott added.
More Key Findings from the 4Q21 Optical Components Report:
- Supply chain difficulties limited Telecom optical components market growth the most in 2021. However, the segment is forecast to grow more than 8% in 2022.
- Consumer component revenue for 3D sensing applications was flat YoY as lower-cost components offset higher unit shipments.
- Industrial optical components used for welding and medical applications grew 18% in 2021, following a weak 2020. Following the acquisition of Coherent, II-VI is poised to control over 50% of this market.
- 1.8M QSFP-DD Datacom modules shipped during 2021, most of which were DR4 format. The report also tracks SR4, FR4, and LR4 Datacom transceivers.
- Over 60k 400Gbps pluggable coherent modules shipped last year, the majority of which were QSFP-DD ZR. The report captures the shipment details of all the emerging derivatives of this format, including ZR, ZR+, 0dB ZR+, and CFP2 based ZR+.
- Shipments of 200Gbps coherent CFP2 modules grew 17% to just over 200k units during 2021 as Chinese OEMs ramp this speed (which is less dependent on western technology) for longer distance metro and long haul applications.
References:
U.S. cybersecurity firms seek tech standards to secure critical infrastructure
A group of cybersecurity companies that specialize in securing critical infrastructure said they’ve formed a lobbying group to push for technological standards among the private sector and government.
The Operational Technology Cybersecurity Coalition said it will directly work with government to share feedback on policy proposals and adopt uniform technological standards for securing places such as pipelines and industrial facilities. Founding members include Claroty Inc, Tenable Holdings Inc, Honeywell International Inc, Nozomi Networks Inc and Forescout Technologies Inc.
Editor’s Note: What is Cybersecurity?
Cybersecurity is a subset of information security which aims to defend an organization’s cloud, networks, computers, and data from unauthorized digital access, attack, or damage by implementing various defense processes, technologies, and practices. With the countless sophisticated threat actors targeting all types of organizations, it’s critical that your IT infrastructure is secured at all times to prevent a full-scale attack on your clouds, networks, or endpoints and risk exposing your company to fines, data losses, and damage to reputation.
………………………………………………………………………………………………………………
The new cybersecurity industry initiative comes as experts have placed increased scrutiny on what’s known as Operational Technology (OT), a broad array of computer systems that monitor and control industrial equipment.
In May, the cybersecurity firm Mandiant Inc warned that compromises against Internet-connected OT devices were on the rise.
“This work is essential to protect our country’s critical infrastructure,” said Jeff Zindel, vice president and general manager for cybersecurity at Honeywell.
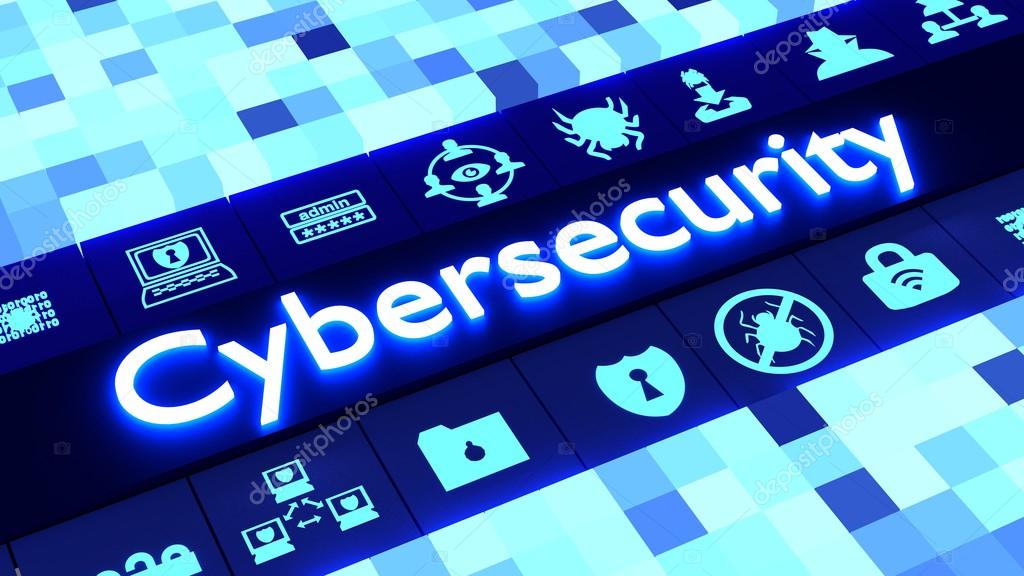
Information Technology (IT) and Operational Technology (OT) are converging, bringing the promise of improved efficiency and new business models enabled by mass digital transformation and the Industrial Internet of Things (IIoT). However, along with the promise of greater connectivity comes greater risk.
As new technologies are introduced and integrated into legacy operations, OT and IT teams are being challenged from every direction. Security approaches that previously worked for one environment may not apply to the other.
That is why a coalition of industry leaders founded the Operational Technology Cyber Security Alliance (OTCSA) — to provide OT operators and suppliers with resources and guidance to mitigate their cyber risk in a fast-evolving world.
An ecosystem approach to safe and secure industrial operations:
The OTCSA is committed to enabling safe and secure operations for the entire OT spectrum. This includes securing the related interfaces to enable interconnectivity to IT while continuing to support and improve the daily life of citizens and workers in an evolving world.
The OTCSA provides OT operators and their vendor ecosystems with regular technical briefs and implementation guidelines to navigate necessary changes, upgrades and integrations. We will build and support an understanding of OT cyber security challenges and solutions from the board room to the factory floor.
The OTCSA adresses cyber security concerns across the entire range of industrial operations, including:
- Industrial control system equipment, software, and networks
- IT equipment and networks that are used in OT systems or provide functionality to OT systems
- Building management systems
- Facilities and control rooms access control systems
- CCTV systems
- Medical equipment
Google’s Topaz subsea cable to link Canada and Japan
The submarine cable system will be the first to link Canada directly to Asia, aiming to provide additional capacity for Google services and to a variety of network operators in Japan and Canada. The cable will be ready for service in 2023.
In August last year, reports were beginning to surface that Canada’s Shaw Communications was building a submarine cable landing station in Port Alberni, on the country’s West coast, despite no new cable systems being formally announced at the time.
It seems that Google was the chairman of this mysterious cable project, announcing the creation of the Topaz system, the first submarine cable set to link Canada directly to Asia. The Topaz cable will take a short hop from Vancouver, Canada, to Port Alberni on Vancouver Island, before travelling West across the Pacific to Japan, where it terminates at the cities of Shima and Takehagi.
In both Port Alberni and Takehagi, landing stations for the cable will need to be built from scratch. Vancouver, on the other hand, having housed the now defunct copper-based Commonwealth Pacific Cable System, built back in 1963, already has a historic landing station available for the project. This facility will be upgraded to meet the needs of the Topaz cable. The final stop, Shima, is already a major hub for submarine cable activity, with three local cable landing stations collectively connecting to around 17 subsea systems.
The Topaz system will comprise 16 fiber pairs with a total capacity of 240 Tbps. It will also make use of Wavelength Selective Switch technology, a software-defined solution that allows greater flexibility when routing traffic on individual fibre pairs.

Google says its intention with the system is not only to allow for low latency access to its own services, like Google Cloud and YouTube, but also to offer capacity for network operators in Canada and Japan.
The company also reportedly intends to swap fiber pair capacity with other submarine cable operators on similar routes, thereby increasing what the company calls “the intercontinental network lattice for network operators”.
Google’s interest and investment in submarine cable projects in recent years cannot be underestimated. The company has announced participation in 20 submarine cable projects, connecting 29 cloud regions, 88 zones, 146 network edge locations across more than 200 countries and territories.
Network infrastructure investments like Topaz bring significant economic activity to the regions where they land. For example, according to a recent Analysys Mason study, Google’s historical and future network infrastructure investments in Japan are forecasted to enable an additional $303 billion (USD) in GDP cumulatively between 2022 and 2026.
The width of a garden hose, the Topaz cable will house 16 fiber pairs, for a total capacity of 240 Terabits per second (not to be confused with TSPs). It includes support for Wavelength Selective Switch (WSS), an efficient and software-defined way to carve up the spectrum on an optical fiber pair for flexibility in routing and advanced resilience. We’re proud to bring WSS to Topaz and to see the technology is being implemented widely across the submarine cable industry.
While Topaz is the first trans-Pacific fiber cable to land on the West Coast of Canada, it’s not the first communication cable to connect to Vancouver Island. In the 1960s, the Commonwealth Pacific Cable System (COMPAC) was a copper undersea cable linking Vancouver with Honolulu (United States), Sydney (Australia), and Auckland (New Zealand), expanding high-quality international phone connectivity. Today, COMPAC is no longer in service but its legacy lives on. The original cable landing station in Vancouver — the facility where COMPAC made landfall on Canadian soil — has been upgraded to fit the needs of modern fiber optics and will house the eastern end of the Topaz cable.
Traditional and treaty rights, and local communities, are deeply important to our infrastructure projects. The Topaz cable is built alongside the traditional territories of the Hupacasath, Maa-nulth, and Tseshaht, and we have consulted with and partnered with these First Nations every step of the way.
“Tseshaht is very proud of this collaboration and our partnership with Google, who has been very respectful and thoughtful in its engagement with our Nation. That’s how we carry ourselves and that’s how we want business to carry themselves in our territory.“ — Tseshaht First Nation – Elected Chief Councillor-Ken Watts
“The five First Nations of the Maa-nulth Treaty Society are pleased that we have concluded an agreement with Google Canada and have consented to the installation of a new, high-speed fiber optic cable through our traditional territories. This agreement, in which both Google Canada and our Nations benefit, is based on respect for our constitutionally protected treaty and aboriginal rights and enhances the process of reconciliation. We would also like to acknowledge the sensitivity that Google Canada expressed during our talks in regard to the pain and trauma experienced by our people as a result of residential school experience. We look forward to a long and mutually beneficial relationship with Google Canada.” —Chief Charlie Cootes, President of the Maa-nulth Treaty Society
“Google’s respect towards our Nation is appreciated and has good energy behind it.” —Hupacasath First Nation – Elected Chief Councilor – Brandy Lauder
With the addition of Topaz, Google has announced investments in 20 subsea cable projects. This includes Curie, Dunant, Equiano, Firmina and Grace Hopper, and consortium cables like Blue, Echo, Havfrue and Raman — all connecting 29 cloud regions, 88 zones, 146 network edge locations across more than 200 countries and territories. Learn about Google Cloud’s network and infrastructure on our website and in the below video.
………………………………………………………………………………………………………
India Telcos say private networks will kill 5G business
India’s top tier wireless network operators have called on the Telecom Regulatory Authority of India (Trai) – India’s telecom sector regulator – to scrap the proposed move to administratively allocate 5G spectrum on demand for private enterprise networks through a publicized online portal-based process, warning that such a step could destroy the 5G business case in the country.
The businesses have mentioned that such a proposal, if accepted by the federal government, might probably rob telcos of their future 5G enterprise revenues – estimated at round 40% of the whole for 5G. Revenue loss to such an extent won’t justify needed capital expenditure in establishing 5G networks, they’ve argued.
In its newest suggestions on 5G spectrum pricing, the Trai has advised that non-public enterprises straight acquire 5G spectrum from the federal government and set up their very own captive wi-fi non-public community (CWPNs). It has additionally beneficial a “gentle contact’ on-line portal-based regime” for buying such permissions/licences for establishing CWPNs. Trai has additionally advised that non-public enterprises have the choice to lease spectrum from telcos to arrange their very own captive non-public 5G networks.
“By permitting non-public captive networks for enterprises, Trai is dramatically altering the trade dynamics and hurting the monetary well being of the telecom trade reasonably than bettering it,” the Mobile Operators Affiliation of India (COAI) said in an announcement Tuesday, April 12th.
The COAI represents Reliance Jio, Bharti Airtel and Vodafone Thought (Vi). It added that “enterprise companies represent 30-40% of the (telecom) trade’s total revenues…non-public networks as soon as once more dis-incentivises the telecom industry to spend money on networks and in addition proceed paying excessive levies and taxes.”
The COAI has urged Trai to revisit its newest suggestions and disallow non-public enterprise networks to make sure monetary viability and orderly progress of the telecom trade that, it mentioned, is greater than able to delivering these companies to companies.
India’s mobile carriers had earlier advised Trai that any proposal to put aside 5G spectrum for personal enterprise networks for captive use both at no cost or at an administrative worth would even be legally untenable.
Trai has beneficial that the spectrum for personal networks be assigned administratively on demand by way of a extensively publicized on-line portal-based course of in a good and clear method. However the regulator has left it to the telecom division to determine whether or not such administrative allocation can be legally tenable or not, based mostly on DoT’s spectrum allocation coverage.
In its 5G dialogue paper, Trai had mentioned the likes of Germany, Finland, UK, Brazil, Australia, Hong Kong and Japan had put aside spectrum within the mmWave band for personal captive 5G networks, whereas Slovenia, Sweden and Korea deliberate to put aside each mmWave and mid-band 5G spectrum for such captive networks.
Subsequently, Tata Communications (TCL), Larsen & Toubro (L&T) and cigarette maker ITC had strongly countered the telcos’ place, and known as on Trai to again earmarking devoted spectrum non-public captive networks and undertake international practices to create a personal 5G ecosystem for enterprises to drive the federal government’s Make in India imaginative and prescient.
However one other high telco government mentioned that if operators’ potential 5G income flows from the profitable enterprise enterprise vertical dry up, they might see little enterprise sense in bidding aggressively for 5G airwaves within the upcoming sale.
Bulk of the bidding urge for food for 5G airwaves, he mentioned, stems from the sturdy income potential of the B2B enterprise enterprise. But when that income stream disappears, telcos gained’t have a viable enterprise case to splurge huge cash on 5G spectrum, particularly as they’ve sufficient spectrum to proceed their current cellular broadband companies operations.”
Know-how corporations, although, have strongly backed Trai’s name to permit non-public enterprises to straight acquire 5G spectrum from the federal government by way of the executive route.
“By way of non-public networks, Trai’s suggestions handle the pursuits of TSPs (telecom service suppliers), enterprises and the general public as extra non-public networks would result in extra employment alternatives and enterprise, and translate into larger financial output and advantages,” mentioned the Broadband India Discussion board (BIF), which counts Cisco, Amazon, Google, Microsoft, Fb-owner Meta, Qualcomm and Intel amongst its key members.
Earmarking unique spectrum for personal 5G networks, it mentioned, would additionally present an “enchancement over common SLAs (service level agreements) of public networks, moreover guaranteeing full lack of interference between them”.
BIF added that Trai’s name for task of spectrum administratively for personal 5G networks “is most acceptable” because it has thought of that captive wi-fi non-public networks should not public networks and don’t have any market prospects, and are restricted to a selected location.”
Trai chairman PD Vaghela termed the 5G spectrum rates “reasonable”, saying they have been arrived at after considering various factors including quantum of spectrum offered, competition in the market and profitability.
……………………………………………………………………………………………………
April 18, 2022 Addendum:
Spectrum users have started squabbling over the telecom regulator’s recommendation to earmark certain bands for captive wireless private networks.
The cellular operators under the COAI have opposed regulator suggestions of allowing enterprises to build their own private 5G networks for captive purposes.
Technology players such as Facebook, Google, Indian Space Association (ISpA) want the 28.5GHz band spectrum to be reserved and allocated exclusively for satellite communications (satcom) services.
The COAI said “by allowing private captive networks for enterprises, telecom regulator Trai is dramatically altering the industry dynamics and hurting the financial health of the industry rather than improving it”.
“Telecom service providers have and going forward will invest lakhs of crore rupees in network rollouts. Enterprise services constitute 30-40 per cent of the industry’s overall revenues. Private networks once again disincentivises the telecom industry to invest in networks and continue paying high levies and taxes.”
The Broadband India Forum (BIF), the industry body of tech players such as Facebook and Google, welcomed the recommendations to allow private networks.
Telcos flay plan for private 5G networks
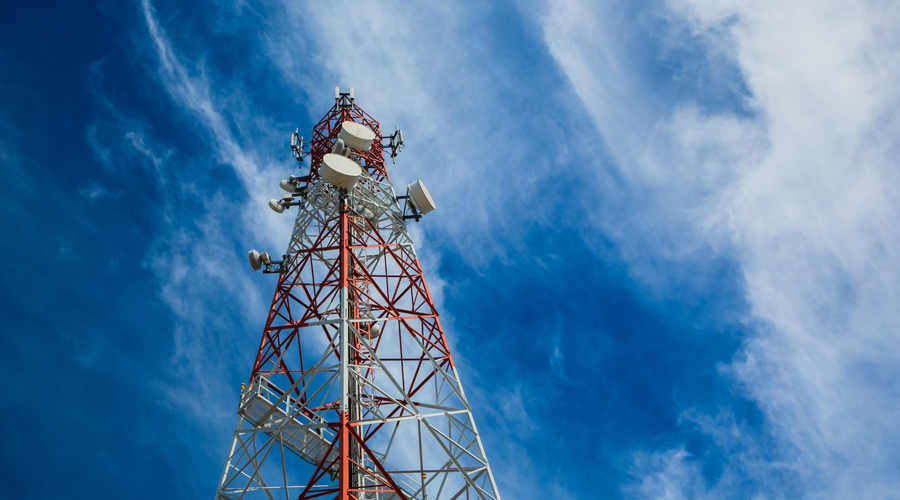
Spectrum users have started squabbling over the telecom regulator’s recommendation to earmark certain bands for captive wireless private networks.
The cellular operators under the COAI have opposed regulator suggestions of allowing enterprises to build their own private 5G networks for captive purposes.
The suggestion has been welcomed by the Broadband India Forum (BIF).
Technology players such as Facebook, Google, Indian Space Association (ISpA) want the 28.5GHz band spectrum to be reserved and allocated exclusively for satellite communications (satcom) services.
The COAI said “by allowing private captive networks for enterprises, telecom regulator Trai is dramatically altering the industry dynamics and hurting the financial health of the industry rather than improving it”.
“Telecom service providers have and going forward will invest lakhs of crore rupees in network rollouts. Enterprise services constitute 30-40 per cent of the industry’s overall revenues. Private networks once again disincentivises the telecom industry to invest in networks and continue paying high levies and taxes.”
The Broadband India Forum (BIF), the industry body of tech players such as Facebook and Google, welcomed the recommendations to allow private networks.
The Indian Space Association (IsPA) said “we welcome Trai’s recommendation that calls for coexistence of satellite communications and IMT in the 27.5-28.5GHz band. We also appreciate recommending an exclusion zone for satellite earth stations in the 27.5-28.5GHz band.”
IsPA opposed the inclusion of all spectrum in the 24.25-28.5GHz bands along with low and mid bands in the auction as it was a case of oversupply to the telecom operators at the cost of the satellite industry.
“The 24.25-27.5GHz bands along with 3.3-3.67GHz bands would be more than sufficient for 5G. Therefore, in line with the global best practices, the 28GHz band should be allocated exclusively for satellite communications,” it added.
In its recent recommendations, telecom regulator Trai said spectrum in three bands – 3700-3800MHz, 4800-4990MHz and 28.5-29.5GHz band – could be earmarked for captive wireless private networks which, it felt, could co-exist with non-IMT services.
In the 28.5-29.5 MHz band, Trai said: “A software based transparent system should be built to permit the establishment of private networks and satellite earth Stations based on the geo-coordinates of the proposed location on interference free co-existence basis.”
The regulator added: “DoT should develop a digital map with geographic coordinates of all the existing and future satellite earth stations as well as geographic coordinates of the premises of Private Network locations. Based on this database, permissions for establishment of new installations may be provided to the licensees.”
https://www.telegraphindia.com/business/telcos-flay-plan-for-private-5g-networks/cid/1861119
References:
https://economictimes.indiatimes.com/topic/telecom-regulatory-authority-of-india
Related: IEEE/SCU SoE Panel Session on Open RAN and 5G Private Networks:
IDC: Cloud Infrastructure Spending +13.5% YoY in 4Q-2021 to $21.1 billion; Forecast CAGR of 12.6% from 2021-2026
According to the International Data Corporation (IDC) Worldwide Quarterly Enterprise Infrastructure Tracker: Buyer and Cloud Deployment, spending on compute and storage infrastructure products for cloud infrastructure, including dedicated and shared environments, increased 13.5% year over year (YoY) in the fourth quarter of 2021 (4Q-2021) to $21.1 billion. This marked the second consecutive quarter of year-over-year growth as supply chain constraints have depleted vendor inventories over the past several quarters. As backlogs continue to grow, pent-up demand bodes well for future growth as long as the economy stays healthy, and supply catches up to demand.
For the full year 2021, cloud infrastructure spending totaled $73.9 billion, up 8.8% over 2020. IDC predicts spending on cloud infrastructure services to increase 21.7% in 2022 to $90.0 billion.
The service provider category includes cloud service providers, digital service providers, communications service providers, and managed service providers. In 4Q21, service providers as a group spent $21.2 billion on compute and storage infrastructure, up 11.6% from 4Q20. This spending accounted for 55.4% of total compute and storage infrastructure spending. For 2021, spending by service providers reached $75.1 billion on 8.5% year over year growth, accounting for 56.2% of total compute and storage infrastructure spending. IDC expects compute and storage spending by service providers to reach $89.1 billion in 2022, growing at 18.7% year over year.
At the regional level, year-over-year spending on cloud infrastructure in 4Q21 increased in most regions.
- Asia/Pacific (excluding Japan and China) (APeJC) grew the most at 59.5% year over year.
- Canada, Central and Eastern Europe, Japan, Middle East and Africa, and China (PRC) all saw double-digit growth in spending.
- The United States grew 5.6%.
- Western Europe and Latin America declined for the quarter.
For 2021, APeJC grew the most at 43.7% year over year. Canada, Central and Eastern Europe, Middle East and Africa, and China all saw double-digit growth in spending. Japan grew in the high single digits, while Western Europe grew in the low single digits. The United States grew 1.5%. Latin America declined for the year. For 2022, cloud infrastructure spending for most regions is expected to grow with the highest growth expected in the United States at 27.8%. Central and Eastern Europe is the only region expected to decline in 2022 with spending forecast to be down 21.7% year over year.
Longer term, IDC expects spending on compute and storage cloud infrastructure to have a compound annual growth rate (CAGR) of 12.6% over the 2021-2026 forecast period, reaching $133.7 billion in 2026 and accounting for 68.6% of total compute and storage infrastructure spend. Shared cloud infrastructure will account for 72.0% of the total cloud amount, growing at a 13.4% CAGR. Spending on dedicated cloud infrastructure will grow at a CAGR of 10.7%. Spending on non-cloud infrastructure will flatten out at a CAGR of 0.5%, reaching $61.2 billion in 2026. Spending by service providers on compute and storage infrastructure is expected to grow at a 11.7% CAGR, reaching $130.6 billion in 2026.
…………………………………………………………………………………………………………………………………………………………………………………………………………………………………………………………………………………………………………….
Separately, Amazon Web Services (AWS) led with 33% of spending on cloud infrastructure services in Q4 2021, according to a Feb 3, 2022 blog post from research group Canalys. Meta, previously known as Facebook, recently chose AWS as a long-term strategic cloud service provider and continues to deepen the relationship as Meta begins to move away from social media to become a broader metaverse company over the next five years. AWS also announced key customer wins across retail, healthcare and financial services and emphasized a key agreement with Nasdaq to migrate markets to AWS to become a cloud-based exchange.
Microsoft Azure was second with 22% of spending, followed by Google Cloud with 9%. The three companies accounted for 64% of total cloud investment for 2021.
…………………………………………………………………………………………………………………………………………………………………………………………………………………………………………………………………………………………………………….
IDC’s Worldwide Quarterly Enterprise Infrastructure Tracker: Buyer and Cloud Deployment is designed to provide clients with a better understanding of what portion of the compute and storage hardware markets are being deployed in cloud environments. The Tracker breaks out each vendors’ revenue into shared and dedicated cloud environments for historical data and provides a five-year forecast. This Tracker is part of the Worldwide Quarterly Enterprise Infrastructure Tracker, which provides a holistic total addressable market view of the four key enabling infrastructure technologies for the datacenter (servers, external enterprise storage systems, and purpose-built appliances: HCI and PBBA).
Taxonomy Notes:
IDC defines cloud services more formally through a checklist of key attributes that an offering must manifest to end users of the service.
Shared cloud services are shared among unrelated enterprises and consumers; open to a largely unrestricted universe of potential users; and designed for a market, not a single enterprise. The shared cloud market includes a variety of services designed to extend or, in some cases, replace IT infrastructure deployed in corporate datacenters; these services in total are called public cloud services. The shared cloud market also includes digital services such as media/content distribution, sharing and search, social media, and e-commerce.
Dedicated cloud services are shared within a single enterprise or an extended enterprise with restrictions on access and level of resource dedication and defined/controlled by the enterprise (and beyond the control available in public cloud offerings); can be onsite or offsite; and can be managed by a third-party or in-house staff. In dedicated cloud that is managed by in-house staff, “vendors (cloud service providers)” are equivalent to the IT departments/shared service departments within enterprises/groups. In this utilization model, where standardized services are jointly used within the enterprise/group, business departments, offices, and employees are the “service users.”
For more information about IDC’s Enterprise Infrastructure Tracker, please contact Lidice Fernandez at [email protected].
References:
https://www.idc.com/getdoc.jsp?containerId=prUS48998722
Gartner: Accelerated Move to Public Cloud to Overtake Traditional IT Spending in 2025
Strong growth for global cloud infrastructure spending by hyperscalers and enterprise customers
Gartner: Global public cloud spending to reach $332.3 billion in 2021; 23.1% YoY increase
Moody’s skeptical on 5G monetization; Heavy Reading: hyperscalers role in MEC and telecom infrastructure
In a recent “Top of Mind” series report, Moody’s said, “The adoption of 5G is gaining momentum. Yet we question how fast companies can roll out 5G and the ability to generate revenue from applications based on 5G technology.”
“We do not expect material revenue increases in the global telecom sector from 5G in the 2022-2025 period. This is because 5G will mainly evolve around enhanced mobile broadband, which will be broadly similar to 4G.”
Wireless network operators have invested heavily in 5G spectrum, network infrastructure upgrades and the credit rating and financial research firm concludes global carriers’ capex will continue to rise through 2025. That’s despite more tepid carrier capex forecasts from Dell’Oro Group and others.
“Global capex growth is expected to moderate from 9 percent in 2021 to 3 percent in 2022, before tapering off in 2023 and 2024,” wrote Stefan Pongratz of Dell’Oro.
Wireless carriers’ capex as a share of revenue leveled off at 16% globally in 2019 and 2020, inched up to 17% in 2021, and is expected to hit 18% for the next four years, according to Moody’s.
Wireless telcos have cumulatively spent $200 billion globally on 5G spectrum to date, according to Moody’s, and GSMA predicts operators will invest about $510 billion on 5G-related infrastructure and services from 2022 to 2025.
…………………………………………………………………………………………………………………..
On the income side of the ledger, wireless carriers have experienced a prolonged period of flat to declining revenue. Rising costs and flat revenue portends a rough four-year stretch for operators, and there’s little to suggest that dynamic will change by 2026. One analyst said the only real revenue generator for wireless telcos in the last few years has been selling their cell towers!
The largely unmet promise of 5G, with no real “killer apps,” follows previous disappointments for carriers in the 3G and 4G time periods. Indeed, they did not make any money of mobile apps, cloud computing/storage, interactive gaming, edge computing or really any value added services.
“This phase carries the greatest uncertainty about companies’ capital spending. As a result, we remain cautious when projecting revenue growth derived from 5G until there is clarity on the business case, especially given the lessons of limited monetization of 4G and 3G,” Moody’s analysts wrote.
Specialized services for enterprises continue to be the most compelling use cases for 5G, and additional IoT applications could drive incremental revenue gains after 2025 but those are unlikely to justify carriers’ significant 5G investments, the financial research firm said.
While ultra low latency might be important (assuming 3GPP release 16 “URLLC in the RAN” spec is completed, performance tested and deployed), the resulting “almost immediate network response time is only relevant in specialized use cases, Indeed, it has become apparent that the most compelling use cases for 5G revolve around businesses rather than residential consumers,” the Moody’s analysts wrote.
“The wide array of potential applications — such as autonomous vehicles, robotics, and smart homes — places different demands on networks in terms of speed and latency, in contrast to previous generations that focused on one major advance, such as broadband mobile video with 4G or web browsing with 3G,” the analysts wrote.
………………………………………………………………………………………………………………..
Moody’s missed a very crucial point related to 5G revenues: that the hyperscalers (Amazon, Microsoft, Google) will get an increasing share of 5G SA core network services and MEC revenues. That’s because of the partnerships wireless carriers have made with the big cloud service providers.
Heavy Reading noted that in a recent blog post. Heavy Reading conducted a survey in collaboration with Accedian, Kontron and Red Hat. The survey questioned 82 communications service providers (CSPs) that have launched edge computing solutions or are planning to do so within 24 months. One of the objectives of the survey was to examine the go-to-market strategies of the CSP and the role the hyperscalers have assumed in those strategies.
Hyperscalers have introduced dedicated edge products and embedded their software stack into operator infrastructure, including Internet of Things (IoT) devices and network gateways. They have introduced products dedicated to the telco market, such as Wavelength from Amazon Web Services (AWS), Azure Edge Zones from Microsoft and Anthos for Telecom from Google Cloud. According to Heavy Reading’s survey results, their efforts have paid off, as CSPs have unquestionably decided to partner with hyperscalers in their multi-access edge computing (MEC) services.
Q: Why do you plan to partner with a hyperscaler to deliver your edge computing? Select up to three. (n=82)
Source: Heavy Reading
…………………………………………………………………………………………………………..
Heavy Reading’s most recent edge computing survey determined that the pivot to improved customer experience is the key goal of edge network deployments and that CSPs must clear new paths to achieve this goal. They must do so by:
- Leaning into automation, particularly in overall lifecycle management.
- Building in comprehensive security protections from the design phase forward.
- Enhancing performance control through automation and AI. CSPs’ growing collaborations with hyperscalers are key to achieving these goals and improving ease of deployment, accelerating time-to-market and enhancing cloud-based security.
Heavy Reading’s survey results show that carriers have committed to edge computing and are progressing rapidly with implementations. The deployment of edge computing brings with it issues of scale and complexity. CSPs are most concerned with overall network performance and security. In fact, those companies that have already deployed the edge have a heightened concern about these issues. They are looking for help from their traditional vendor and integrator partners, from their network monitoring and assurance tools and from the hyperscalers.
References:
China’s state policy: shape global tech standards to increase influence and enhance global reputation
In line with China President Xi Jinping‘s goal of making the country a ‘major power with pioneering global influence’ by 2049, China has been leveraging its technological prowess and geopolitical heft to shape the global technological environment and standards to serve its commercial and strategic interests, a media report said.
China has adopted a state-directed strategy to influence international standards setting, and use them as a foreign policy tool to enhance its global standing, the Times of Israel reported, adding that, the Xi administration has employed a dual-track approach to set the international technological standards.
On the one hand, it seeks to influence both the multilateral (governmental) and the multi-stakeholder technical Standards Development Organizations (SDOs) by placing Chinese nationals in senior leadership positions (like in 3GPP and ITU-R WP5D) and larger representation of Chinese tech companies (the three China state owned network providers, Huawei, ZTE and smaller players like Nufront with its own 5G RIT spec), and other Chinese companies, with guidance from the Party-State (CCP), are creating standards utilizing the Belt and Road Initiative (BRI) and Digital Silk Road (DSR).
With respect to 5G radio interface technology (RIT) standards, three China ministries (MIIT, NDRC and MOST) jointly established the “IMT-2020(5G) Promotion Group” in February 2013. The objectives have been met:
– Promote the development of 5G technologies in China.
– Facilitate cooperation with foreign companies and organizations MIIT Ministry of Industry and Information Technology.
– Drive China’s contributions to ITU-R WP5D 5G standards (M.2150 and revisions of M.1036) and 3GPP release 15 and 16 specifications (a Chinese national heads up the critical 3GPP “URLLC in the RAN” project).
The Group helped progress the ITU-R M.2150 standard for 5G RAN/RIT/SRITs. Initially China had it’s own 5G RIT spec, but it was later merged with 3GPPs as was South Korea’s.
At the International Telecommunications Union (ITU), the involvement of Chinese commercial entities have increased after the impetus provided by ITU‘s current Secretary-General, Zhao Houlin who has served two terms as Director of ITU’s Telecommunication Standardization Bureau (STB). China is second only to the U.S. in the number of entities registered as ITU members, according to the referenced Times of Israel blog.
In 2021 alone, Chinese entities backed 145 new standards at the ITU, up from 46 in 2015 and six times more than Western entities.
The number of Chinese nationals in secretariat and leadership positions in critical multi-stakeholder SSOs such as the International Organization for Standardization (ISO) and the International Electro-technical Commission (IEC) has also surged in the past decade.
Beyond the IEC, ISO, and ITU, Chinese actors are also active in other SDOs including the 3rd Generation Partnership Project (3GPP) that develops 5G technical specifications, as well as Internet Engineering Task Force (IETF), the report said, adding that the companies such as Alibaba, Baidu, Huawei, Tencent and ZTE are advanced members of the IEEE Standards Association.
Not only do Chinese firms ‘flood’ committees with a huge volume of standards proposals and contributions, but they also typically vote as a single bloc. Beijing also has a tendency to use its debt and trade leverage to influence the votes of a number of countries in favour of its proposals. This produces a strikingly high rate of success in the number of Chinese submissions at the ITU, the Times of Israel blog noted.
Another emerging facet of China’s approach to technology standards setting is the Digital Silk Road (DSR) initiative, which is one of the primary vehicles delivering Chinese technology to BRI partner states. By signing agreements with BRI partner governments, Beijing is propagating its own technology standards in project host states, creating dependencies that lock these countries into using Chinese vendors and standards.
Beijing’s moves are aimed at setting global standards for the next-generation technologies, the report said, adding, that it wants to gain control over key technologies like the Internet of Things, Cloud Computing, Big Data, 5G and artificial intelligence.
In light of above, it is apparent that Beijing’s moves are aimed at setting global standards for the next-generation technologies. The CCP wants to gain control over key technologies like Internet of Things (IoT), Cloud Computing, Big Data, 5G and artificial intelligence.
International organizations need to be wary of these maneuvers in order to prevent Beijing from dominating global technology standards and thus gaining a monopoly over the world’s future-shaping technologies, the Times of Israel report concluded.
References:
https://blogs.timesofisrael.com/double-standards-chinas-influence-in-international-standards/
https://www.ifri.org/sites/default/files/atoms/files/seaman_china_standardization_2020.pdf
https://techblog.comsoc.org/2018/11/18/with-no-5g-standard-imt-2020-china-is-working-on-6g/
https://techblog.comsoc.org/tag/chinas-imt-2020-promotion-group/
5G tech to improve health care access in Zimbabwe; new cyber risks require “zero trust” policies from telcos
Speaking at the ICT conference in the capital of Harare yesterday, Zimbabwe Information Communication Technology minister Jenfan Muswere said the 5G technology promises to provide essential levels of connectivity to enable a new health ecosystem.
“In telemedicine today, we can consult a doctor anywhere in the world through a video call over the Internet. But the connection is still not good enough for that doctor to perform surgery remotely. 5G will change that. One that can meet patients and provide needs accurately, efficiently, conveniently, cost-effectively and at substantial scale. (Note that 5G will only improve the access over 3G/4G, but not the metro or core network performance which could be a bottleneck).
This will lead to faster medical services and better patient outcomes. The new and reliable network also means that large files can be transmitted quickly between doctors and hospitals.
“The reduction in time that 5G brings means more timely diagnostics, second opinions, treatment starts and adjustments since the medical data can be transmitted and consumed by doctors faster than ever before, whether at home or in the office,” Muswere said.
Jenfan Muswere, Zimbabwe ICT Minister
………………………………………………………………………………………………………………………….
The ICT Minister added that Zimbabwe’s economic resilience and competitiveness lay in digitalization, hence the need for the adoption of emerging technologies.
“According to the Global System for Mobile Communications (GSMA), the number of global Internet of Things (IoT) connections between 2019 and 2025 will more than double to almost 25 billion. New products and services in IoT, as well as other areas like autonomous vehicles and robotics, and augmented reality will create new revenue streams that are expected to add USD 2.2 trillion to the global economy by 2034.
For businesses and industry, 5G and IoT will provide a wealth of data allowing them to gain insights into their operations like never before. Businesses will operate and make key decisions driven by data, innovate in agriculture, smart farms and manufacturing, paving the way for cost savings, better customer experience and long-term growth,” Muswere said.
The minister further said the country is already making significant strides in its digital transformation journey but service providers should tighten cyber security as it is vulnerable to crime.
“We have already developed a 5-Year Strategic Plan that promises to revolutionize government services and bring connectivity to under-served rural communities.”
Telecom operators under the ministry have already started rolling out 5G network, which are quite fast, with peak data rates of up to 100 times faster than 4G-LTE networks. 5G will support ultra low latency, once the 3GPP Release 16 URLLC in the RAN spec has been completed and performance tested. That will minimize 5G RAN delay in sending and receiving information between devices.
“However, as we expand the adoption of 5G, we must be mindful of the potential for new cyber risks. Consumers and businesses must have confidence that our 5G networks are resilient. With that in mind, telecom operators must commit to adopt a ‘zero-trust’ posture. Telecom operators must first verify all activity before trusting it. There must also be constant monitoring and vigilance for suspicious activities,” Muswere concluded.
…………………………………………………………………………………………………………………………………………………………….
This comes as Zimbabwe is moving towards embracing 5G to facilitate a competitive digital economy. Econet Wireless, one of the few network providers in Zimbabwe, launched a 5G network earlier this year using Ericsson network equipment. Ericsson will support Econet with its latest energy-efficient and high-performing Radio Access Network (RAN) and 5G Evolved Packet Core (EPC) solutions for 5G deployment in the capital Harare. The solutions will boost Econet’s capacity and user experience with significantly faster network speeds, which are up to 10 times the speed of 4G technology.
Econet CEO Douglas Mboweni said: “Working with Ericsson to launch 5G in Zimbabwe is an important milestone in our digital transformation journey. As we deploy the network across the country, our goal remains that of meeting and addressing our customers’ needs using the latest technology available. We are certain that Ericsson’s industry-leading and energy-efficient 5G solutions will help us achieve that goal, and significantly improve our customer experience through reliable connectivity to our subscribers.”
Todd Ashton, Vice President and Head of Ericsson South and East Africa said: “We continue to strengthen our long-term partnership with Econet by providing Ericsson’s latest technology in Zimbabwe. 5G will play a critical role in accelerating the digitalization of the economy, increase financial inclusion and stimulate next-generation innovation for consumers and enterprises in the country. With our commitment to enhancing the digital infrastructure on the continent, we look forward to accelerating Zimbabwe’s digital future together with Econet and bring the benefits to the entire continent of Africa and in line with our #AfricaInMotion campaign, which focuses on empowering a connected and sustainable Africa.”
…………………………………………………………………………………………………………………………………………..
References:
https://www.ericsson.com/en/press-releases/1/2022/econet-and-ericsson-launch-5g-in-zimbabwe